Mohanad Albughdadi
AI & Remote Sensing Specialist | Google Cloud ML Engineer | Python Expert
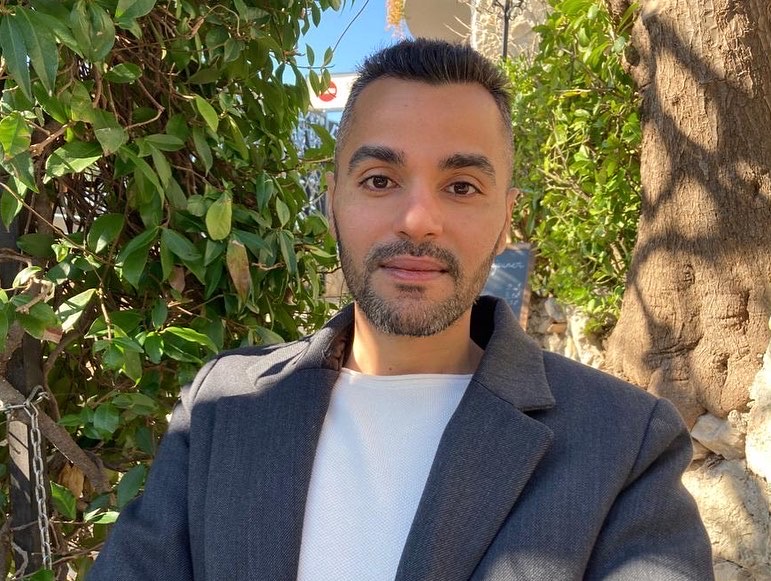
Use CLAY embeddings to detect changes caused of floods using Sentinel-1 and Sentinel-2 data.
Deploy and manage STAC catalog components using a simple Docker Compose setup.
Super resolution of Sentinel-2 to the resolution of CERRA data.
Super resolution of ERA-5 temperature to the resolution of CERRA data.
Super resolution of ERA-5 windspeed to the resolution of CERRA data.
An API based on Docker, Kubernetes, Celery, Redis and Flower to download and process Sentinel-2 images.
OBB YOLOv8 object detection from VHR remote sensing images.
Interactive segmentationb dashboard of Sentinel-2 images using SAM.
Finetune SAM foundation model for downstream tasks in remote sensing.
Deep learning-based approach to automate the extraction of field boundaries for precision agriculture.
Enhancing spatial resolution for Sentinel-2 images to 2.5m.
Mapping fire risk zones through deep learning and satellite imagery to aid disaster management.
Fast and efficient estimation of Leaf Area Index (LAI) for large areas using deep learning.
Leveraging deep learning to detect swimming pools across French communes, supporting circular economy efforts.